Addressing AI misconceptions for educators: 5 concepts to wrap your head around
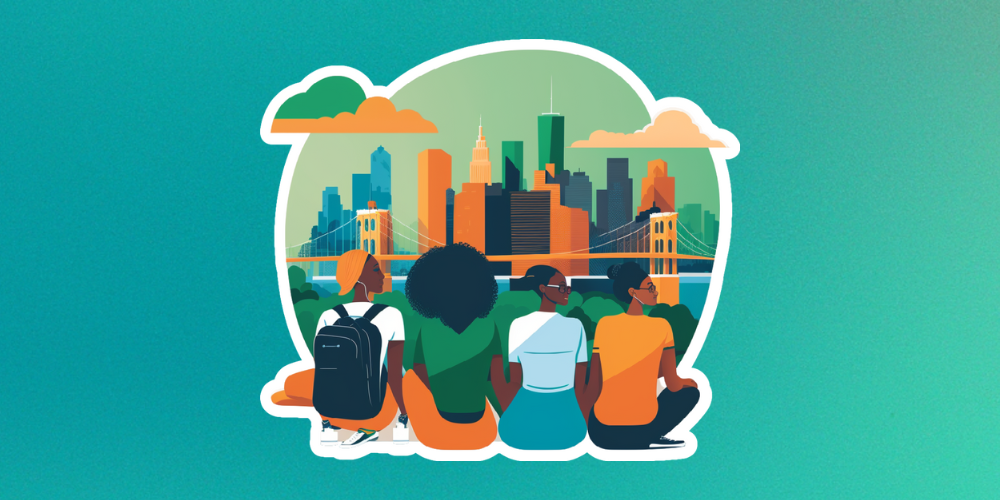
It feels like just about every day that there’s some new announcement of an artificial intelligence (AI) breakthrough that’s going to change the world. That’s both amazingly exciting and also just the tiniest bit overwhelming. At Mindjoy, we’ve been helping educators and students navigate these changes, and we’ve learned a lot that we can share with you.
As with any technological change, it’s only natural that misconceptions crop up from time to time. We’d like to help frame these ideas in simple terms, so you can join the conversation with confidence. At the end of the day, we believe that educators and students need to be involved in order to help shape the future.
For the purposes of today, we’re mostly going to be talking about a relatively new form of AI called “generative AI”, aptly named due to its surprising ability to generate new data given an input. Although these new techniques have become popular recently through products like chatGPT, they build on much older technologies that you’re already familiar with. Think Google Translate or Siri! You’ll also see references to Large Language Models (LLMs), which are essentially just AI models that are trained on really large amounts of data in order to generate human-like language. Let’s jump in!
AI is magic!
While we agree it’s magical, it’s not quite magic per se. As with most things (including magic tricks themselves), the appearance of magic is usually because you don’t have a good idea of what’s happening behind the scenes. One of our favourite examples to show educators is using GPT-3 to complete a song. For example, consider “Uptown Funk” by Mark Ronson and Bruno Mars. If we were to input the first line of the song, the the model successfully completes the next line for us:
> This hit, that ice cold
< Michelle Pfeiffer, that white gold
If we remove more words and leave just a few hints, it’s still remarkably good at completing the next line:
> This hit,
< that ice-cold, Michelle Pfeiffer, that white gold
However, if we remove the final comma, there’s just not enough context for the model to identify the song:
> This hit
< me like a ton of bricks.
Indeed, GPT-3, indeed. These examples show you what’s happening behind it all – the model is completing your inputs. Specifically, it’s predicting which word should appear next given the previous series of words (and repeating this many times over). While this produces some truly astounding results and involves some really complex calculations, it’s actually somewhat intuitive.
AI just said the darndest thing....
With the concept of completions in mind, it’s worth considering our role as humans interacting with AI. In this early stage of generative AI, we’ve seen our fair share of funny and unexpected outputs from models. A fairly common response to these mishaps is that the model is broken, buggy or unreliable. And while all of these things may be true, it’s worth casting your thoughts back to what it is you’re actually doing: asking an AI model to complete your input.
So if the completed text is faulty… could it be that your input has a fault in it too? Oftentimes, this is the case. We haven’t given enough context or information for the model to give us what we’re looking for. Or maybe we’ve even caused some confusion ourselves! This type of interaction with AI is often referred to as “human in the loop”. The model generates some output, and you’re able to make changes or guide the AI to make improvements. In this sense, it’s useful to think of yourself as a conductor: you’re directing the AI to some conclusion, and it absolutely needs your input to get there. You’re on stage taking the glory, but you’re also responsible for the success of the show!
ChatGPT learns while we’re talking?
Since these new AI models are so good at generating text, it often appears like they’re learning while they’re talking to you. In fact, we’ve heard this question from a number of educators over the last few months. It turns out that with the current generation of AI models, this is not the case. GPT actually stands for Generative Pretrained Transformer – the operative term being “pretrained”. These models are trained on such unfathomably large datasets that the computation needs to be completed with the help of specialised hardware and even sometimes supercomputers. Not to mention that it takes a really really long time to get it all done.
Alas, there is some nuance here. Although these models are typically pretrained, some do allow for “fine-tuning”, which just means that you can help guide them towards particular outputs by showing some examples. Not to mention that Bing is testing an AI chatbot that can search the internet while you’re chatting with it.
This being said, at least for now, you can rest easy with the knowledge that you’re learning more from your conversation than the AI is!
The model knows details about me and my friends
Well well, somebody’s famous! If this is in fact true, then there is a distinct possibility that one of the popular Large Language Models was trained on data that mentions you. As a result, it would know about you (and possibly your friends). But for those of us that aren’t in the limelight, it’s quite likely that you’re reading more into the output of the model than is actually there.
A similar effect is well studied in psychology, known as the Barnum effect. It’s the phenomenon of individuals placing high accuracy on descriptions of their personality despite the fact that these descriptions are rather general and vague. We all know the feeling of reading your horoscope and thinking that it simply must have been written for you! This despite the fact that we read it in a newspaper produced for thousands of readers.
AI might be having a similar effect on us, and it’s worth keeping that in mind when you discover an output that’s eerily close to home.
The Large Language Model is plagiarising!
A topic on the minds of many educators at the moment is academic integrity in the age of AI. Part of this is our definition of plagiarism, which historically has implied the unreferenced usage of another person’s original work. Large Language Models do in fact produce original outputs that have never been seen before, although they are trained on original works of humans. So now that software can do this, it looks like our definition may need to be updated!
We’re also seeing some debate over the idea of what makes an original work in the first place – is AI-generated content original if the AI was trained on other humans’ original work? At the moment, there’s a lawsuit attempting to answer this question in relation to the code-generating AI assistant by Github.
Recently, the International Baccalaureate schools announced that they would not ban AI in schools, and that they will require that AI-generated content is cited and quoted. They don’t believe that banning technology is an effective way of dealing with innovation, and these new tools will become part of our everyday lives. At Mindjoy, we really like this framing. AI is going to be a part of the future, so how can we adapt education to meet the challenge? If students are able to use AI to complete their homework in a few minutes, is this the right homework to be setting in the first place? What kinds of skills do students need in a world where AI can generate content in record time?
The key point here is that things are nuanced in this new world, and we need to adapt our definitions and goals to meet the challenge.
Takeaways
To end off, we’d like to leave you with a few questions you can ask yourself when working with Large Language Models:
- How can I better understand the underlying mechanisms of an AI model so that I understand the outputs?
- What strategies can I use to ensure that the inputs I provide to an LLM achieve my desired outcomes?
- How can I recognize when I’m reading more into the model’s output than is actually there, and what does that teach me about my own thinking?
Hopefully this discussion helps you frame conversations around AI in the best way possible. The future is really exciting, and we need educators and students to be a part of the conversation.